The nascent regulatory landscape of web-scraping
We consider the extent to which regulatory and enforcement bodies have regulated web-scraping
Nov 2, 2023
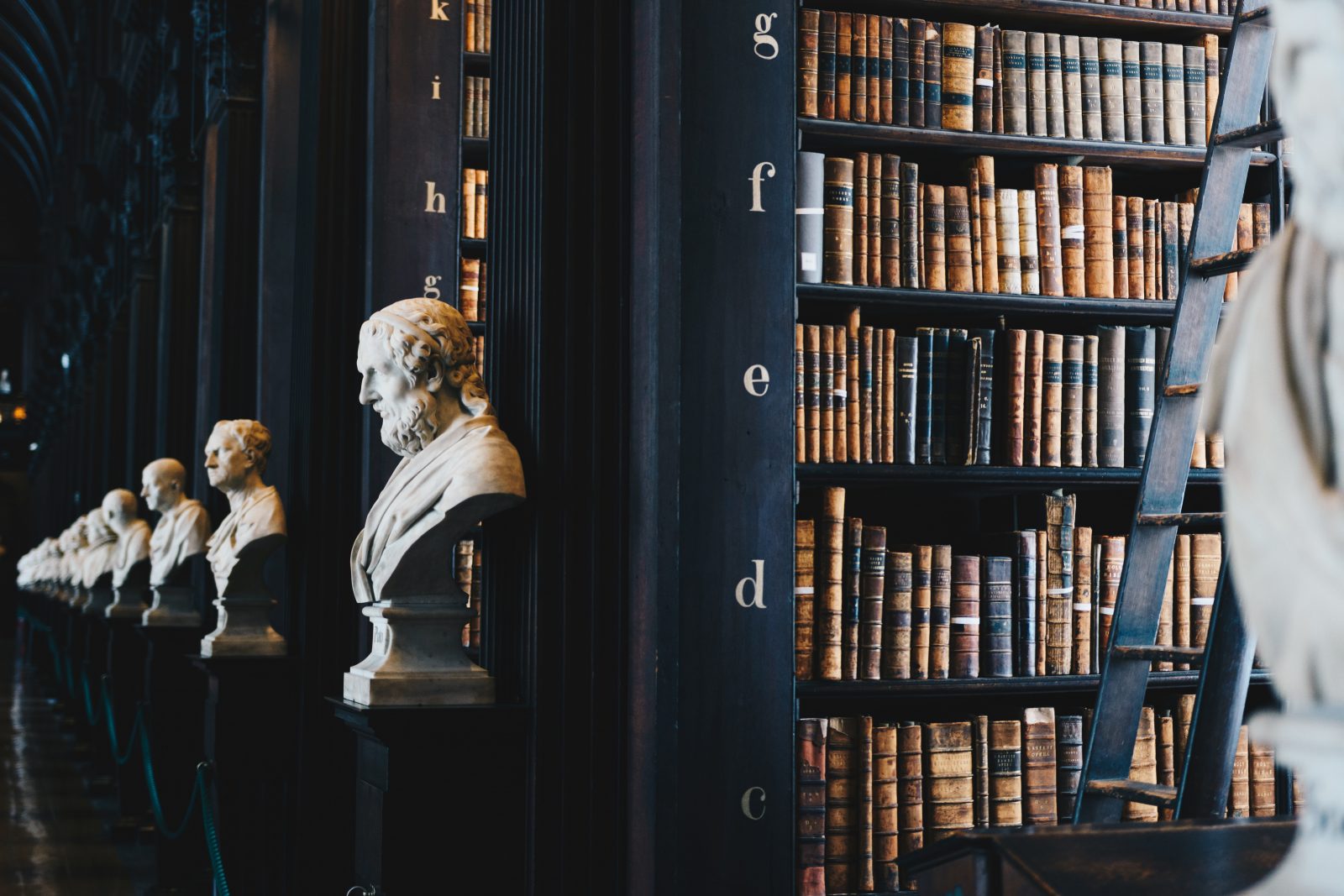
Web-scraping, sometimes called data extraction or aggregation, is the practice of collecting vast amounts of data available online through automated means. The data aggregation industry recently has exploded as companies rapidly learn the competitive advantage that big data may bring, including in the verticals of generative AI (with AI systems trained on scraped data) and investment decisions (often informed by valuable big data).
Hedge funds, in particular, have made significant use of scraped data. One research report suggests that in 2018, one out of every 20 website visits came from a fund or sell-side research firm gathering data. These web-scraping efforts come alongside hedge funds’ existing practices of purchasing aggregated data sets from data collectors, sometimes with exclusivity clauses guaranteeing that no other hedge fund will gain access to the data set.
In this article, we consider the limited extent to which regulatory and enforcement bodies have regulated web-scraping and predict areas that these regulators may be tuned into in the not-so-distant future.
The Securities and Exchange Commission
This summer, the SEC took an initial step toward regulating the use of predictive data analytics by broker-dealers and investment advisers through a proposed rule regarding conflicts of interest.
The SEC’s request for comment focuses on the use of data analytics by broker-dealers and advisers, rather than on the processes through which data is collected and aggregated by web-scrapers. Nevertheless, the proposed rule may suggest heightened SEC scrutiny of data collection, aggregation, and use in general, which may implicate web-scrapers in the future.
One emerging issue, which the SEC may well explore, is whether reliance on scraped data for investment decisions could amount to insider trading. Insider trading generally takes two forms, each “addressing efforts to capitalize on nonpublic information through the purchase or sale of securities.” The “classical theory” involves a corporate insider trading in the securities of his or her corporation on the basis of material, non-public information—i.e., information regarding the corporation that is not “available to the public through SEC filings, the media, or other sources.” The “misappropriation theory” of insider trading, meanwhile, extends liability to anyone (including those who are not corporate insiders) who trades on the basis of confidential information belonging to a securities issuer in breach of a duty owed to the source of the information.
Although the SEC’s Division of Enforcement is likely giving thought to potential actions for insider trading stemming from the broad-based use of AI to aggregate data to effectively trade on that information, such potential causes of action do not fit neatly within existing legal standards governing insider trading liability under Section 10(b) and Rule 10b-5 of the Exchange Act.
First, data collected through scraping is typically publicly available, and trades based on public information are not historically subject to insider trading liability. Nevertheless, some commentators have posited that public data—once scraped, aggregated, processed, and analyzed—could amount to more than the sum of its parts and convey information that is, in fact, non-public. Although individual data points may indeed be public, trends or aggregated statistics derived from a larger collection of data may not be publicly available or easy to ascertain.
In this vein, the European Union’s securities regulator recently updated its regulations to counsel market actors who trade using “[r]esearch and estimates based on publicly available data” to “consider the extent to which the information is non-public and the possible effect on financial instruments traded in advance of its publication or distribution,” which a commentator has theorized could subject trades based on scraped data to insider trading liability.
Whether or not the commentator’s interpretation is valid, in the United States neither Congress nor the SEC has made any comparable effort to broaden the scope of information that is considered non-public for insider trading purposes. Instead, existing case law makes clear that information can be public even if it is “known only by a few securities analysts or professional investors.”
Given that the vast majority of hedge funds specialize in analyzing large sets of public information to inform their investment decisions, any move toward deeming aggregated public data non-public because it is known only to a small community of investors would have significant implications, essentially creating a risk of liability for an investor’s skill in estimating the profitability of any given trade.
Second, establishing a trader’s liability for insider trading under the “misappropriation theory,” described above, requires a showing that the inside information was obtained in breach of a fiduciary duty, a requirement that will often be difficult to satisfy in the context of arms-length data providers regardless of whether they are offering publicly available or private information.
The SEC and DOJ can potentially overcome this hurdle in cases in which scraped data is obtained unlawfully, however, as opposed to lawful use of data aggregation tools to compile public information, as evidenced by the handful of hack-to-trade cases the SEC and criminal agencies have prosecuted.
The seminal hack-to-trade case is SEC v. Dorozhko, in which the Second Circuit determined that the use of computer hacking to obtain confidential insider information could attract insider trading liability, even if the hacker was not in a fiduciary relationship with the source of the data.
In that case, the defendant was alleged to have hacked into a web platform that housed the earnings reports of IMS Health, Inc., a public issuer, hours before those reports were released to the public. His trades based on his alleged advanced access to the earnings reports resulted in a net profit of almost $300,000. Having determined that hacking could constitute a fraudulent misrepresentation and thereby attract insider trading liability even in the absence of a fiduciary relationship, the Second Circuit remanded the case for further consideration of whether the hacking at issue involved the use of misrepresentations to gain access to the confidential information. The defendant was later ordered to forfeit his profits. Other courts have applied the reasoning in Dorozhko to cases involving similar facts.
None of these cases has implicated the typical bread and butter of data scrapers, however, which involves the aggregation of large collections of publicly-available data, rather than advance access to specific, highly sensitive non-public data, such as the contents of press releases.
One commentator has theorized that the scraping of data in violation of a website’s terms of service also potentially could amount to a breach of fiduciary duty or deceptive behavior in contravention of the securities laws, but concluded that “[e]nforcing the terms of use of a public domain is not the job of Section 10(b) and Rule 10b-5, but rather a task to be brought about by ordinary contract and tort law.”
A market manipulation case involving the use of scraped data is easier to imagine, but similarly unlikely to succeed. Market manipulation involves conduct, including trading, “designed to deceive or defraud investors by controlling or artificially affecting the price of securities.”
Given the growing practice of hedge funds using non-public data sets, often obtained through scraping technology, the SEC could conceivably charge a case in which an investor timed its trades to take advantage of future market movement, as predicted through the use of non-public scraped data.
Existing jurisprudence, however, makes clear that as long as the investor disclosed its reliance on a data set, the principle that “[t]he market is not misled when a transaction’s terms are fully disclosed” is likely to defeat any charge of market manipulation.
The Federal Trade Commission
In August 2022, the Federal Trade Commission published an advance notice of proposed rulemaking that requested public comment on “whether it should implement new trade regulation rules or other regulatory alternatives concerning the ways in which companies collect, aggregate, protect, use, analyze, and retain consumer data, as well as transfer, share, sell, or otherwise monetize that data in ways that are unfair or deceptive.” The FTC appears primarily focused on curtailing businesses’ data collection practices and increasing data security safeguards to prevent problems like identity fraud, which can result from data breaches.
It is likely that any future regulation by the FTC on data could alter the existing data landscape with far-reaching consequences for data scrapers—and action by the agency appears increasingly imminent.
First, data scrapers may face greater challenges in pursuing their scraping activities if the FTC passes rules in this area. The FTC’s advanced notice has emphasized possible rulemaking in the area of “data security,” which the Commission has defined as “breach risk mitigation, data management and retention, data minimization, and breach notification and disclosure practices.”
If future rules require businesses to ensure the data they store is kept out of reach of third parties, scrapers may encounter obstacles in accessing or aggregating data that was previously more accessible.
Similarly, proponents of increased FTC regulation in this area have emphasized the concept of data minimization, which requires that “data should only be collected, used, or disclosed as reasonably necessary to provide the service requested by a consumer.” If enforced through FTC rule or regulation, a policy of data minimization may well limit the scope and volume of data available for harvesting by web-scrapers, in addition to limiting the data that scrapers are permitted to collect.
Second, future regulation by the FTC may also impose restrictions on the use of data, which would affect scrapers that sell aggregated data sets to third parties.
Regulatory agencies in foreign countries have already enacted such use restrictions. For instance, in April 2020, the French Supervisory Authority published regulations regarding the use of publicly available data for marketing purposes, requiring companies that scrape personal data to first seek users’ consent and emphasizing that an individual’s decision to share data with one business does not entail a grant of permission to a third party to also use that data. Widespread regulations governing the use of scraped data in the United States could similarly impact web-scrapers’ business practices.
And lastly, it is possible that any FTC rules or regulations regarding the collection, storage, or sale of data—although intended to target businesses that gather customer data while providing other goods or services—could also capture and apply to businesses like web-scrapers whose sole purpose is to collect data.
Privacy Regulations
Although data privacy has not been the subject of any federal regulation in the United States, several states and foreign jurisdictions have enacted laws and regulations that protect data privacy. Data scrapers must ensure their compliance with all such applicable data privacy regimes.
In the United States, the most expansive privacy regime is the California Consumer Privacy Act, signed into law in 2018, and further expanded in 2020 by the California Privacy Rights Act. These laws give California residents the right to know which personal information is collected and stored by businesses operating in California, combined with a right to demand that any such personal information be deleted. These provisions mirror many codified in the European Union’s General Data Protection Regulation (the “GDPR”), which protects the personal information of people within E.U. member states and purports to govern the processing of all data regarding European “data subjects.”
Failure to adhere to the requirements of privacy regimes, including the GDPR, has come with hefty fines for technology companies. Most notably, Clearview AI, which runs a facial recognition database, has been ordered to pay almost $22 million in fines to Italy’s privacy authorities and more than £7.5 million to the UK’s privacy authorities. Other jurisdictions, such as Canada and France, have ordered Clearview AI to delete data concerning their residents.
Data scrapers could be subject to similar fines if they deal with personal information in ways prohibited by the applicable privacy regulations.
Employment Regulators
There have been few other attempts to regulate data scraping among other federal agencies, but regulators of all types remain attentive to the growing use of big data in society.
For instance, the Equal Employment Opportunity Commission held a panel in 2016 to consider the implications of big data on hiring processes and employment discrimination. Panelists discussed, among others, the ways in which data analytics can help employers “develop a set of characteristics of high-performing incumbents, and, through the use of wide-ranging and potentially disparate data points, match candidates for the position with those desired profiles.”
But panelists were wary that the relevant data sets might directly or indirectly cause employers to discriminate against candidates from protected groups—for instance, by screening candidates with disabilities and thereby contravening antidiscrimination laws.
Although the EEOC’s press releases refer expressly to data scraping, the Commission appears more focused on the use of data rather than on the process by which it is obtained, and any future regulation from the Commission is therefore not likely to impact web-scraping.
Renita Sharma, Hope Skibitsky, and Daniel Sisgoreo are experienced litigators in Quinn Emanuel’s New York office. They have extensive experience representing clients in the data scraping space, including on issues relating to the intersection of data scraping/aggregation and the Computer Fraud and Abuse Act, violations of terms of service and related torts. They also frequently advise clients on ways to mitigate their legal risk profile with respect to data collection and usage.
1 Bradley Saacks, Hedge funds are watching a key lawsuit involving LinkedIn to see if they can spend billions on web-scraped data, Business Insider (Mar. 14, 2019, 1:48 PM), https://www.businessinsider.com/hedge-funds-watching-linkedin-lawsuit-on-web-scraped-data-2019-3
2 See, generally, Ian Allison, Big Data, Big Problem: Could Wall Street See Insider Trading Lawsuits over Selling Data Sets?, Newsweek (Oct. 11, 2017, 8:25 AM), https://www.newsweek.com/could-wall-street-see-first-legal-action-selling-data-sets-682188
3 Conflicts of Interest Associated with the Use of Predictive Data Analytics by Broker-Dealers and Investment Advisers, 88 Fed. Reg. 53960 (Aug. 9, 2023).
4 U.S. v. O’Hagan, 521 U.S. 642, 653 (1997).
5 Id. at 651-52.
6 U.S. v. Contorinis, 692 F.3d 136, 143 (2d Cir. 2012); see also SEC v. Mayhew, 121 F.3d 44, 50-51 (2d Cir. 1997).
7 O’Hagan, 521 U.S. at 652.
8 See, generally, Florian N. Kamp, Alternative Data Accumulation, Investment Management and the Ever-Present Spectre of Insider Trading Liability—Should Hedge Funds be Concerned about Trading on Scraped Data?, 22 U. Pa. J. Bus. L. 627.
9 Id., at 642 (“While the emphasis on the public origin of the scraped data has some superficial appeal, it seems more sensible to qualify the aggregated data set as a new piece of information that was not previously available. In today’s ubiquity of data, purposefully gathering data and distilling it to a set that actually conveys a message to the surveyor that the amorphous array of material were not (yet) able to articulate, is a skill so material that it warrants classifying the aggregation as a novel piece of information.”).
10 Regulation (EU) No 596/2014 of the European Parliament and of the Council of 16 April 2014 on market abuse (market abuse regulation) and repealing Directive 2003/6/EC of the European Parliament and of the Council and Commission Directives 2003/124/EC, 2003/125/EC and 2004/72/EC, 2014 O.J. (L 173) 1, 7-8; see Kamp, supra note 8, at 643.
11 Contorinis, 692 F.3d at 143.
12 574 F.3d 42 (2d Cir. 2009).
13 Ukrainian hacker liable in SEC insider trading case, Reuters (Mar. 29, 2010, 5:24 PM), https://www.reuters.com/article/us-sec-hacker/ukrainian-hacker-liable-in-sec-insider-trading-case-idUKTRE62S5DH20100329
14 See, e.g., U.S. v. Khalupsky, 5 F.4th 279 (2d Cir. 2021); U.S. v. Klyushin, 2022 WL 17983984 (D. Mass. Dec. 2, 2022).
15 Kamp, supra note 8, at 654.
16 Ernst & Ernst v. Hochfelder, 425 U.S. 185, 199 (1976).
17 Wilson v. Merrill Lynch & Co., Inc., 671 F.3d 120, 130 (2d Cir. 2011) (“In order for market activity to be manipulative, that conduct must involve misrepresentation or nondisclosure.”).
18 Trade Regulation Rule on Commercial Surveillance and Data Security, 87 Fed. Reg. 51273 (Aug. 22, 2022).
19 Cristiano Lima, FTC consumer protection chief puts data brokers on notice, The Washington Post (Sept. 21, 2023, 9:00 AM), https://www.washingtonpost.com/politics/2023/09/21/ftc-consumer-protection-chief-puts-data-brokers-notice/
20 Id. at 51277.
21 How the FTC Can Mandate Data Minimization Through a Section 5 Unfairness Rulemaking, Consumer Reports & Electronic Privacy Information Center (Jan. 26, 2022), https://advocacy.consumerreports.org/wp-content/uploads/2022/01/CR_Epic_FTCDataMinimization_012522_VF_.pdf
22 La réutilisation des données publiquement accessibles en ligne à des fins de démarchage commercial, Commission Nationale de l’Informatique et des Libertés (April 30, 2020), https://www.cnil.fr/fr/la-reutilisation-des-donnees-publiquement-accessibles-en-ligne-des-fins-de-demarchage-commercial
23 The GDPR forms part of the United Kingdom’s domestic law through the Data Protection Act 2018.
24 Kevin Townsend, Facial Recognition Firm Clearview AI Fined $9.4 Million by UK Regulator, SecurityWeek (May 23, 2022), https://www.securityweek.com/facial-recognition-firm-clearview-ai-fined-94-million-uk-regulator/
25 Id.
26 Use of Big Data Has Implications for Equal Employment Opportunity, Panel Tells EEOC, U.S. Equal Employment Opportunity Commission (Oct. 13, 2016), https://www.eeoc.gov/newsroom/use-big-data-has-implications-equal-employment-opportunity-panel-tells-eeoc
Newsletter sign up