Strategic obfuscation: How does anonymised data hold value?
What is anonymised or obfuscated data? What are the data monetisation use cases for obfuscated data?
Nov 28, 2024

In today's data-driven landscape, organisations are constantly looking for new ways to extract more value from the data they generate during their daily operations. This value can come from using the data internally to enhance strategies or selling it as a product to external parties. However, many businesses still have reservations about selling their data because of concerns about disclosure of trade secrets, customer and supplier privacy, and reputational risk.
For example, a company’s data assets may contain personal data that comes within the remit of data protection laws such as the General Data Protection Regulation (GDPR) and California Consumer Privacy Act (CCPA). Each of these laws creates implications for the sale of such data, presenting a complex series of challenges and risks to data monetisation.
In addition, datasets may include sensitive business information that organisations do not wish to share externally. This is particularly common when information relates to third parties such as clients, suppliers, or partners. As a result, the prospective seller may be concerned that the sale of this data would risk business relationships.
Despite these common concerns, accessible methods exist to mitigate risks and maintain the value of data for sale.
Once a decision has been made to sell, license or share an organisation's data with third parties, the next step is to evaluate what can be shared from a regulatory and compliance perspective and what the organisation is comfortable sharing. For many companies there is a sliding scale of value to be considered. In most cases, anonymising or aggregating a dataset will impact its value to the data consumer, with less granularity associated with less value. Nevertheless, an anonymised dataset can still command substantial prices from the right groups of data buyers.
For this document, we use the following definitions:
- Obfuscation makes data unintelligible or hard to use but keeps it somewhat linked to its original form, allowing for potential reversal.
- Anonymisation ensures that the data cannot be traced back to any individual, usually by irreversibly removing personal identifiers.
In this post, we introduce several data anonymisation and obfuscation methods and provide use cases for maintaining value for potential data buyers. We also look at some risks associated with a poorly executed obfuscation strategy. But let's start with a brief explanation of the concept by defining data anonymisation.
Data anonymisation
Anonymisation is an umbrella term that describes any type of operation that removes data or converts it into a form that:
- Theoretically makes it impossible to determine the actual underlying information
- Cannot be reverse-engineered to reidentify the original version
An organisation will typically anonymise personal data as part of its security and compliance responsibilities. Once anonymised, any such data no longer comes within the scope of the GDPR and, by and large, most other data privacy regulations.
However, data anonymisation goes beyond the domain of personal data and serves a valuable role in protecting any kind of sensitive data.
Types of anonymisation
In this section, we break down different types of data anonymisation/obfuscation and, through examples, show how applying these methods can offer value to data consumers in the investment community.
Partial masking, redaction, nulling and attribute suppression
= A range of loosely related data obfuscation methods that withhold identifying information. Partial masking and redaction render data meaningless by concealing some or all of the information. By contrast, data nulling and attribute suppression do so by either replacing data with null values or deleting part of a dataset altogether.
Use case
A company provides a navigation app for taxi users and rideshare passengers to check that their driver is taking an optimal route. This can generate valuable data about the use of different services and for predicting revenues accordingly.
However, the data includes recurring departure points and destinations, which can reveal the personal addresses of app users. To prevent the identification of individuals, the company uses one of these methods to obfuscate pick-up and arrival points in residential areas. Yet, it still offers value by providing journey distances and commercial addresses of driver ports of call.
Synthetic data
Synthetic data is an advanced data obfuscation method that algorithmically generates an artificial version of a real dataset while retaining the original characteristics of the source data. It remains valid as it simulates the patterns in the original data but without any sensitive or confidential content. Currently, the technique is mainly used for artificial intelligence (AI) training.
Use case
A smart meter provider who doesn't have the contractual rights to sell usage data collected from customers, identifies a data monetisation opportunity that doesn't breach its agreement.
It discovers it can obfuscate real usage data using software that creates a synthetic equivalent. The company can then sell the resulting product to investors and utility providers looking to track different types of energy usage, such as gas or electric heating, as they relate to different energy consumers.
Hashing and tokenisation
Hashing is a one-way cryptographic process that converts text into a meaningless fixed-length string of characters. Tokenisation, on the other hand, is a reversible process that replaces data with a token that maps back to the original value using a tokenisation system.
Although hashing and tokenisation are different types of data obfuscation, both methods offer the scope to preserve the relationship between correlative data.
Use case
A credit card company is looking for ways to monetise its data and needs to obfuscate personal data to protect cardholders' privacy.
It decides to replace identifiers with hash values, as this still allows data consumers to track the spending patterns of individuals through their transactions with different merchants. This provides value by showing how merchants attract similar types of customers.
Aggregation
Aggregation combines information from different data points to provide summary details such as totals and averages. Organisations typically use aggregation to make it easier to query and analyse data. However, by nature, it is also a data obfuscation technique.
Data binning is an alternative form of aggregation that groups sensitive data into broader categories, such as time or date intervals, geographical regions or number ranges.
Use case
A company provides a cloud-based resource to help freight carriers analyse their orders. Its dataset details the volumes of different commodities being sent and received by subscribers to its service.
However, it is reluctant to sell the data in its current form, as this would disclose the identity and activity of those companies using its platform. To address the issue, it aggregates shipments to the level of country import and export. The resulting product is useful for potential buyers looking for insight into import and export trends and related macroeconomic indicators.
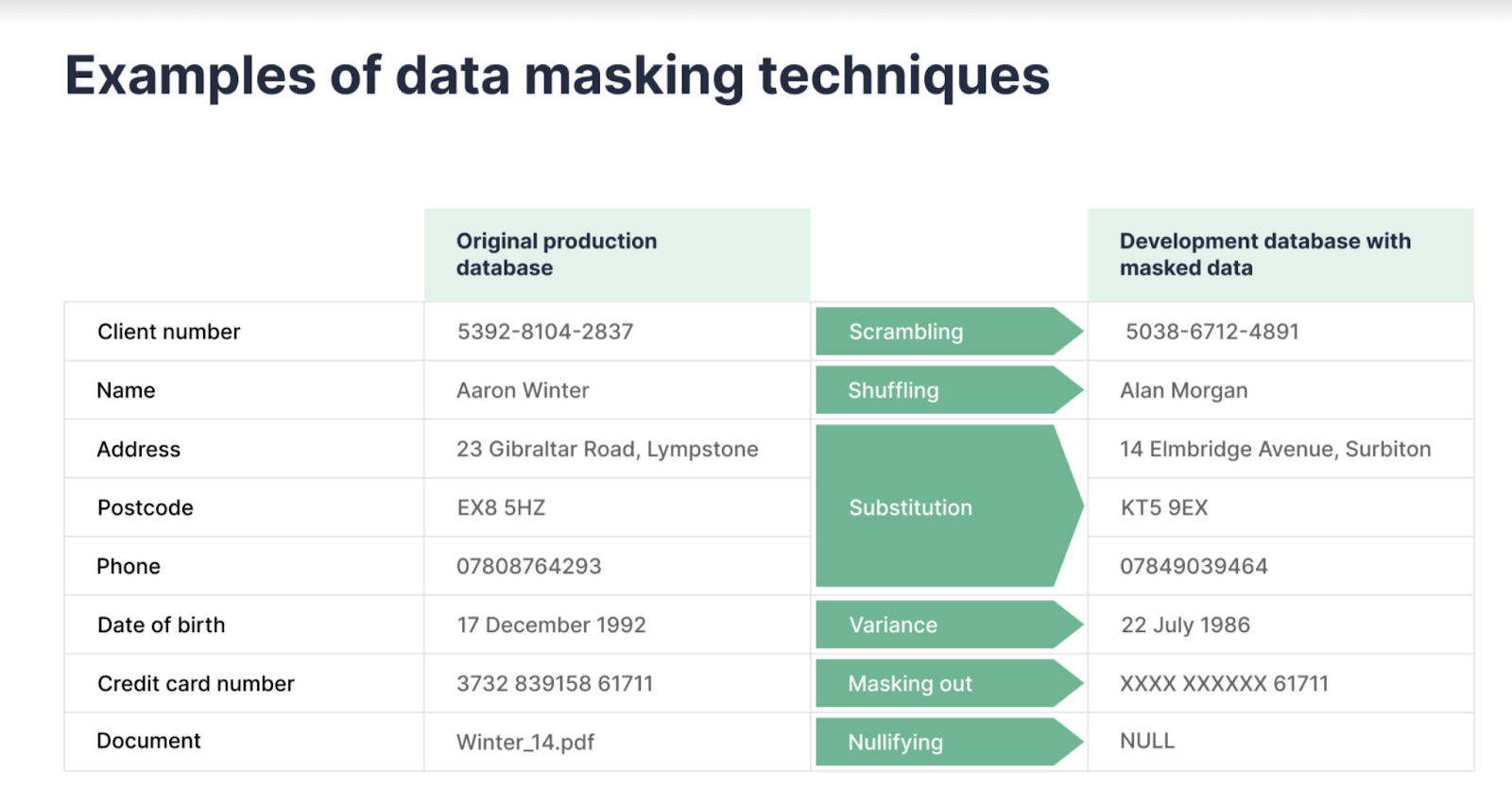
Data monetisation expertise
Data obfuscation can help you address the risks of selling data and overcome your privacy concerns. But how do you know which method best fits your data? How do you implement it correctly to prevent risks to compliance and business relationships? How do you know what data is suitable for monetisation? And how do you market your data product to potential data buyers?
A data monetisation consultancy service can help you answer these questions and highlight potential issues you never anticipated.
Your data could be a vast, untapped resource that investors and other data consumers need. Neudata’s team is well-positioned to help you understand the value of your data. For more information, visit our website or contact us.
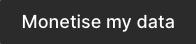